Chapter 4 Targeting
One of the key trends in the advertising industry is (digital) data-driven marketing. The whole thing starts with massive, passive data collection. No matter which website we visit or which app we use: We leave a digital footprint. These footprints are compiled for individual users and form so-called user profiles. A set of similar profiles is then aggregated to user segments, which aim at describing a homogeneous set of users with similar preferences and interests. In order to improve marketing activities, companies use these user segments to show them advertisements fitting the user’s interest. To be more concrete; users visiting car sites are more likely to see BWM or Daimler-Benz ads.
So far this sounds great. It is a win-win situation, advertisers reach an audience with an interest in their product and users see ads that mirror their interest. Well the key is; advertisers want to increase their advertising effectiveness. Let’s have a look at how advertising is measured. Let’s exemplify it by looking at the advertising for a car with two segments; a segment of car enthusiasts and a general population segment. Both segments receive the same ad.
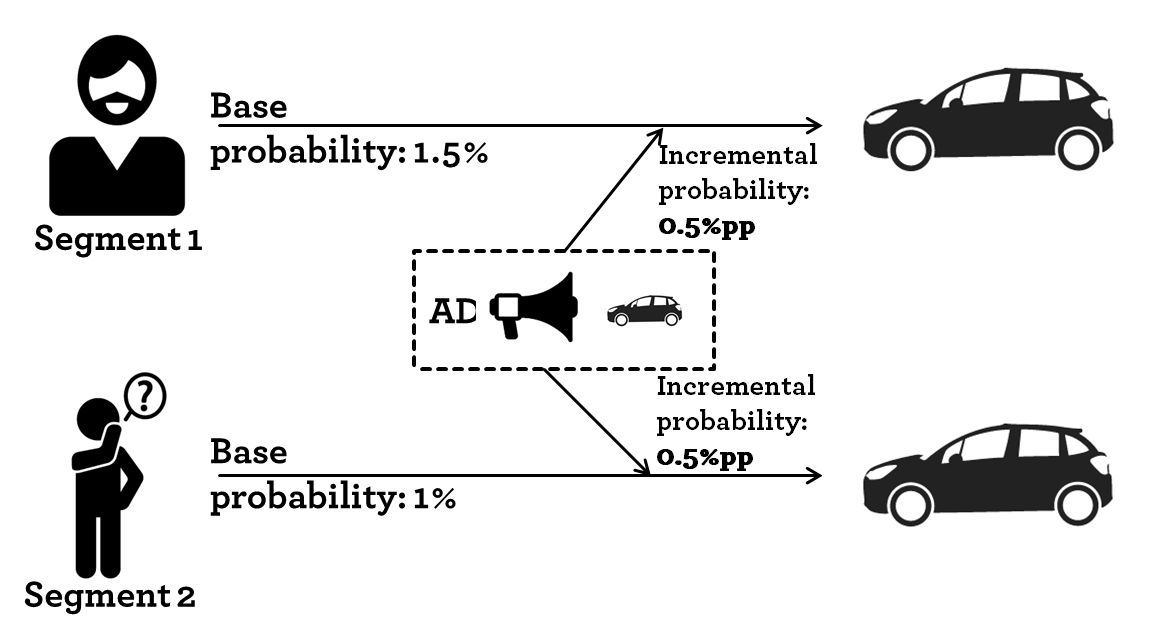
An example of two user segments
The most common (and maybe intuitive) way to look at advertising effectiveness is by calculating the cost per order/conversion. The calculation is straight forward. The cost per order is: the ad spend by user segment divided by the number of orders generated in the user segment.
As segments are generated depending on the user interests, it is fair to assume that users have different base probabilities of buying the item (car) in question.
User segment | #User | Base probability | Ad uplift | Ad Spend | #Orders | Cost per order |
---|---|---|---|---|---|---|
Car enthusiast | 100 | 1.5% | 0.50%pp | 10 000 € | 2 | 5 000 € |
No Targeting | 100 | 1.0% | 0.50%pp | 10 000 € | 1.5 | 6 667 € |
Given the example, a naive marketeer concludes that targeting the car enthusiast segment is more effective as the cost per order is 1 667€ lower.
However that is only the easy way of calculating it. What you as a marketeer really want to measure is the incremental uplift. The uplift describes the increase in purchase probability when showing the user ads. The incremental uplift is only measurable by running (controlled) experiments, in which you measure the increase of sales in the segment exposed to ads to the “same” segment not exposed to ads. Such controlled experiments are rarely done on a segment basis.
In this example, both marketing activities have the same incremental uplift in purchase probability (0.5%pp). Hence, if you ignore that the marketeer have to buy targeting data on top of her media spend, the incremental uplift is the same for both groups as is the return for the ad investment.
This brings us to the key message; current systems (ad serving in particular) are designed in a way that they are basically unable to capture users base purchase probability. Hence, they are deeply flawed and purposefully biased towards benefiting targeted ad buying.
4.1 Targeting - a look at ad effectiveness
In the last chapter, I discussed how the current digital measurement approach is biased towards targeted ad buying. The key reason is that ad effectiveness is calculated on a cost per order/conversion basis. As particular user segments -which are addressed with digital targeting- have a high base purchase probability, the segment looks more responsive. Due to insufficient experimentation and proper measurement, the budget optimization tries to reach users who are likely to order/“convert” anyway. Hence, it ignores incremental effects and instead focuses on overall purchase probabilities, mixing base purchase probability and ad-driven incremental effects.
Let’s go back a step and have a look at two scenarios. Scenario A is taken from the previous post. There are two user segments; a segment of car enthusiasts and a general population segment. Both segments receive the same ad. The car enthusiasts (Segment 1) has a higher base probability of purchasing a car. As discussed that leads to a lower cost per order for that group and sub-sequentially to a misguided budget shift.
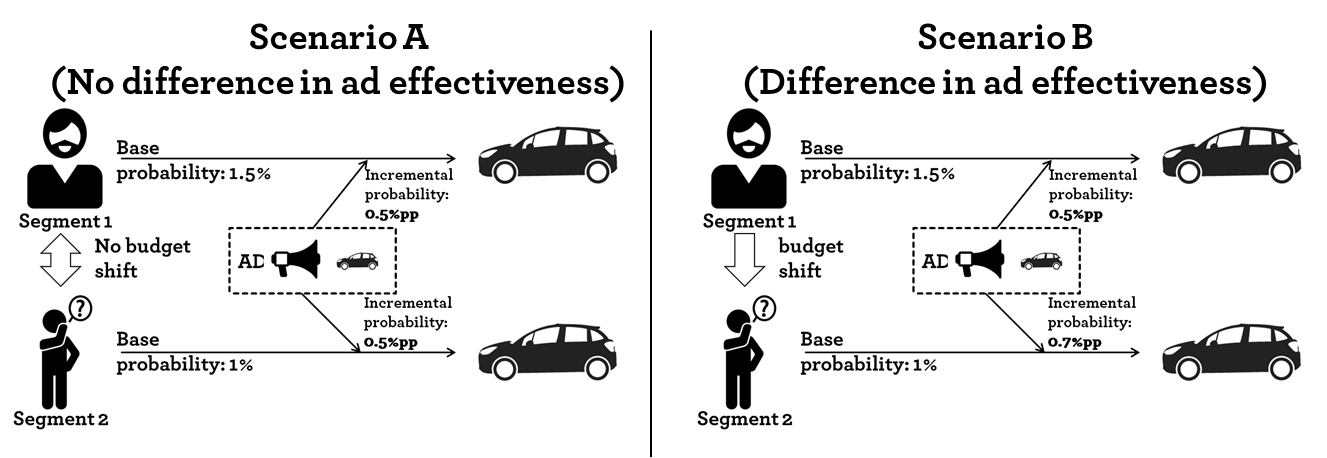
Example of ad effectiveness
Let’s add the second Scenario B. In this case the incremental effect of showing an ad is higher in the the general population segment (Segment 2) compared so Segment 1. It might be that the ad communicates a new feature (Matrix LED lights, head-up-display,…) that Segment 1 was not aware of before. In that scenario a budget shift would increase the overall sales. However, that budget shift would only be realized if the incremental effect would have been measured. The cost per order is still lower for Segment 1.
To sum up, current systems try hard to minimize the cost per conversion. This leads to showing ads to users who are likely to convert anyway, if possible. From a theoretical point of view, (e.g. assuming that targeting works perfectly) that leads to an overall decreasing ad effectiveness.
4.2 Targeting - exemplified with Google Adwords
In the last two chapters, I tried to discuss how measurement and false metrics drive optimization towards low hanging fruits and in the end degrade ad effectiveness. I would like to follow up with a a short example of how the issue extends into the paid search (e.g. Google Adwords) channel.
Search traffic is split up into two parts; the organic, free traffic and paid traffic. The following chart illustrates the difference.
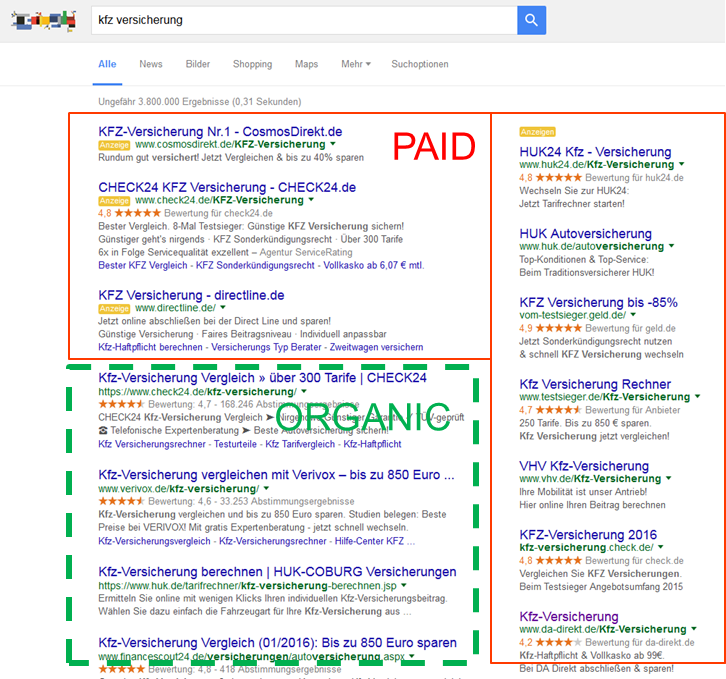
Paid and orangic search traffic
For the paid part advertisers are allowed buy individual keywords (search terms that users might type in) on a cost per click basis. Each keyword sells for a different cost per click depending on competition, relevancy and click-through-rate. Usually the advertiser picks the keywords with lowest cost per click.
Keywords can be clustered into at least two broad categories: Brand and Generic keywords. Brand keywords contain the brand name of the advertiser while generic keywords do not and just relate to an (unspecific) inquiry. From a consumer journey perspective, generic terms are searched in the beginning of a purchase decision process, while brand keywords are used towards the end. Generally, that means that brand keywords have a much lower cost per click than generic keywords. Hence advertisers buy brand keywords all the time.
4.3 Targeting - the increment matters
It is save to assume that advertisers are organically listed in the top positions for brand keywords, while they might not even appear on the first results page for generic terms. As paid advertising is shown above the organic section a majority of users click on the paid links. Hence, an advertiser buying her “own” brand keywords is cannibalizing her organic, free traffic with paid search traffic.
The following chart illustrates this:
The share of incremental traffic for buying generic keywords is much higher compared to buying brand keywords. Using proper experimentation one can figure out what that share is and calculate the cost per incremental visitor as a more reliable metric.
The questions arises why this is usually not done. I see three main reasons: (i) incentives, (ii) additional work and (iii) Google’s quality score.
For the team managing paid search it is much easier to communicate low costs per click (CPC) readily delivered by the system compared to relying on a derived metric that needs constant experimentation. As most people are familiar with CPCs they are also commonly used to benchmark channels and/or team performance. Hence increasing CPCs is a difficult story to tell. A part of the cost per click on a keyword is determined by the quality score, Google assigns to an individual advertiser. The lower the score the higher the cost an advertiser has to pay per click. Even though Google does not openly discuss the algorithm behind the quality score, it is pretty clear that the Click-through-Rate (which is higher for brand keywords) improves the score. As a result of their policy brands are forced or at least given a decent incentive to buy their brand keywords.
To sum up, in the paid search channel, the system is designed in a way to ensure that brands buy keywords for users who are very likely to click/convert anyways.